Background
Language modeling — that is, predicting the probability of a word in a sentence — is a fundamental task in natural language processing. It is used in many NLP applications such as autocomplete, spelling correction, or text generation.
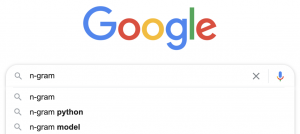
Currently, language models based on neural networks, especially transformers, are the state of the art: they predict very accurately a word in a sentence based on surrounding words. However, in this project, I will revisit the most classic language model: the n-gram models.
- In part 1 of the project, I will introduce the unigram model i.e. model based on single words. Then, I will demonstrate the challenge of predicting the probability of “unknown” words — words the model hasn’t been trained on — and the Laplace smoothing method to alleviate this problem. I will then show that this smoothing method can be viewed as model interpolation, which is the combination of different models together.
- In part 2 of the project, I will introduce higher n-gram models and show the effect of model interpolation of these n-gram models.
- In part 3 of the project, aside from interpolating the n-gram models with equal proportions, I will use the expectation-maximization algorithm to find the best weights to combine these models.
Data
In this project, my training data set — appropriately called train — is “A Game of Thrones”, the first book in the George R. R. Martin fantasy series that inspired the popular TV show of the same name.
Then, I will use two evaluating texts for our language model:
- The first text, called dev1, is the book “A Clash of Kings”, the second book in the same series as the training text. The word dev stands for development, as we will later use these texts to fine-tune our language model.
- The second text, called dev2, is the book “Gone with the Wind”, a 1939 historical fiction book set in the American South, written by Margaret Mitchell. This book is selected to show how our language model will fare when evaluated on a text that is very different from its training text.
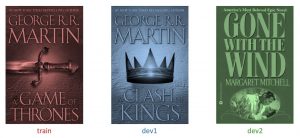
Unigram language model
What is a unigram?
In natural language processing, an n-gram is a sequence of n words. For example, “statistics” is a unigram (n = 1), “machine learning” is a bigram (n = 2), “natural language processing” is a trigram (n = 3), and so on. For longer n-grams, people just use their lengths to identify them, such as 4-gram, 5-gram, and so on. In this part of the project, we will focus only on language models based on unigrams i.e. single words.
Training the model
A language model estimates the probability of a word in a sentence, typically based on the words that have come before it. For example, for the sentence “I have a dream”, our goal is to estimate the probability of each word in the sentence based on the previous words in the same sentence:
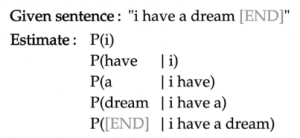
The unigram language model makes the following assumptions: